Image Segmentation in Machine Learning
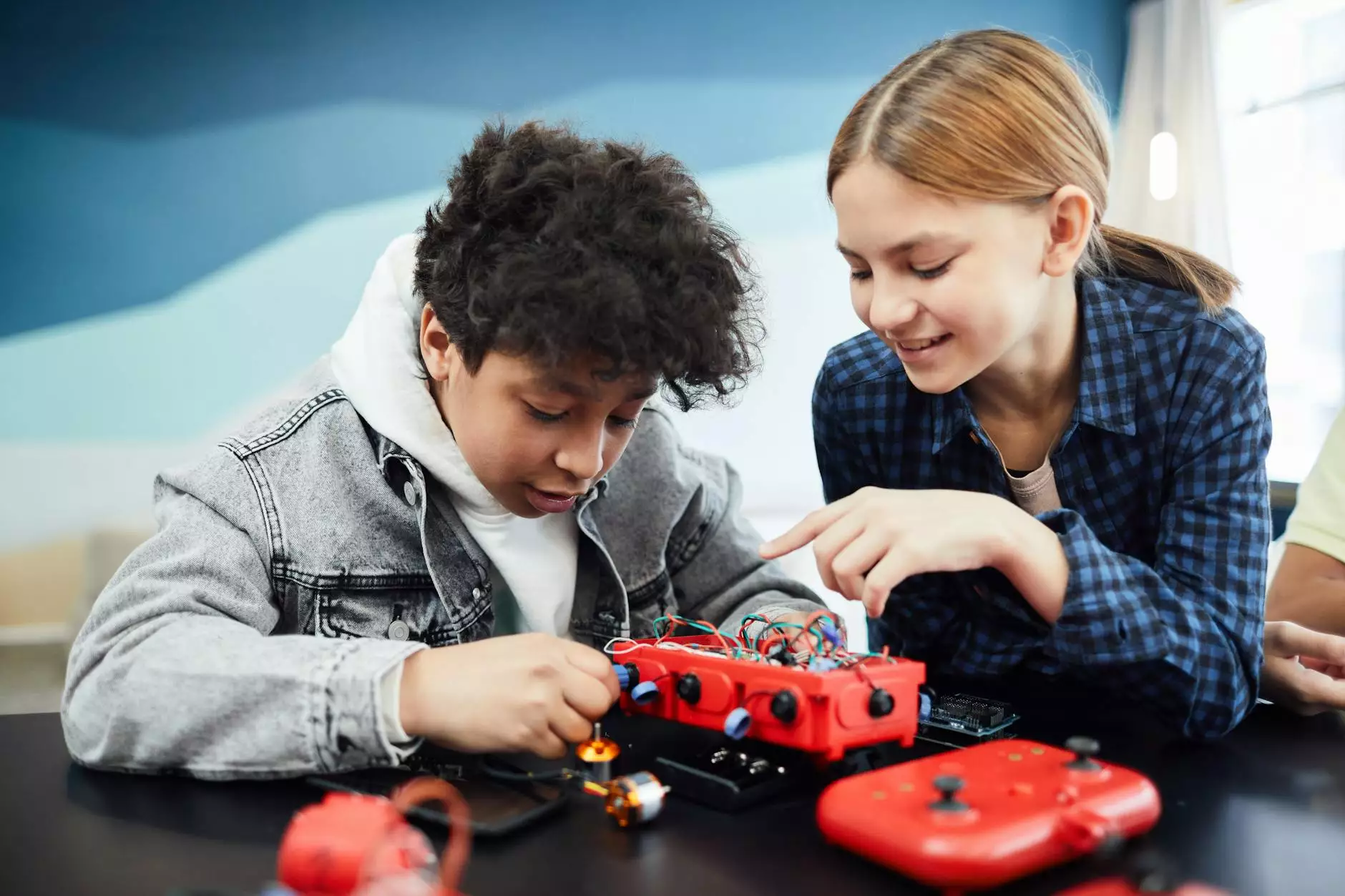
Welcome to Keymakr.com, your trusted source for home services and locksmith expertise. In this article, we will dive deep into the fascinating world of image segmentation in machine learning. Image segmentation plays a crucial role in various industries, and understanding its applications can empower businesses to unlock new possibilities.
The Basics of Image Segmentation
Image segmentation, a fundamental concept in machine learning, involves partitioning an image into meaningful segments or regions. These segments help machines identify and differentiate objects within an image, ultimately aiding in object detection, recognition, and analysis.
One popular technique for image segmentation is Machine Learning-based Image Segmentation. By training a machine learning model on a vast amount of labeled images, it learns to predict the segmentation of new images accurately. This technique has revolutionized computer vision applications, enabling machines to understand visual data with remarkable precision.
Applications of Image Segmentation in Various Industries
1. Medical Imaging
The field of medical imaging heavily relies on image segmentation. By accurately segmenting medical images, doctors and researchers can identify and analyze specific areas of interest, such as tumors or blood vessels. Image segmentation plays a vital role in disease diagnosis, treatment planning, and monitoring the progress of medical interventions.
2. Autonomous Vehicles
Autonomous vehicles heavily rely on computer vision systems that utilize image segmentation to identify and understand the environment. By segmenting the various objects present in the scene, such as pedestrians, vehicles, and traffic signs, autonomous vehicles can make informed decisions and navigate safely on the roads.
3. Security and Surveillance
Image segmentation is essential in security and surveillance systems to detect and track objects of interest. By precisely delineating objects, such as suspicious individuals or unauthorized vehicles, these systems can trigger alerts and help security personnel take proactive measures.
4. Robotics
Image segmentation is also widely used in robotics applications. It allows robots to perceive their surroundings, recognize objects, and interact with them effectively. From industrial automation to household assistance robots, image segmentation helps robots understand complex environments and perform tasks with increased accuracy.
Benefits of Implementing Image Segmentation
Implementing image segmentation offers several benefits to businesses and industries:
- Improved Object Detection and Recognition: Image segmentation enables machines to accurately identify and differentiate objects within an image, leading to enhanced object detection and recognition capabilities.
- Precise Analysis: By segmenting images, businesses can perform focused and targeted analysis on specific regions, enabling better decision-making and insights.
- Enhanced Efficiency: Image segmentation allows automation and optimization of various processes by efficiently separating and isolating objects of interest within an image.
- Enhanced Safety & Security: By utilizing image segmentation, businesses can enhance safety measures, detect anomalies promptly, and prevent potential security risks.
Tips for Successful Image Segmentation Implementation
To maximize the benefits of image segmentation, consider the following tips:
- Choose the Right Algorithm: Different image segmentation algorithms are suitable for different types of images and tasks. Analyze your requirements and select an algorithm that best suits your needs.
- Train the Model with Sufficient Data: Training a model with a diverse and well-labeled dataset is crucial for accurate segmentation results.
- Regularly Update and Fine-Tune the Model: As new data becomes available, continue retraining and updating the model to improve its performance and adapt to evolving conditions.
- Consider Preprocessing Techniques: Preprocessing techniques like noise reduction, contrast enhancement, and image normalization can significantly improve segmentation results.
- Evaluate and Validate Results: Regularly evaluate the segmentation results against ground truth data to measure accuracy and identify areas for improvement.
Conclusion
Image segmentation is a powerful technique in machine learning that enables businesses to unlock valuable insights from visual data. Whether it's in the field of medical imaging, autonomous vehicles, security and surveillance, or robotics, the applications of image segmentation are vast and rapidly expanding.
To achieve successful image segmentation implementation, businesses must choose the right algorithms, train models with sufficient data, regularly update and fine-tune the models, and apply appropriate preprocessing techniques. By following these best practices, businesses can harness the full potential of image segmentation and gain a competitive edge in their respective industries.
At Keymakr.com, we stay at the forefront of technological advancements, ensuring our services are equipped with the latest innovations. Contact us today to learn how our expertise can contribute to your image segmentation needs and other home services.
image segmentation ml